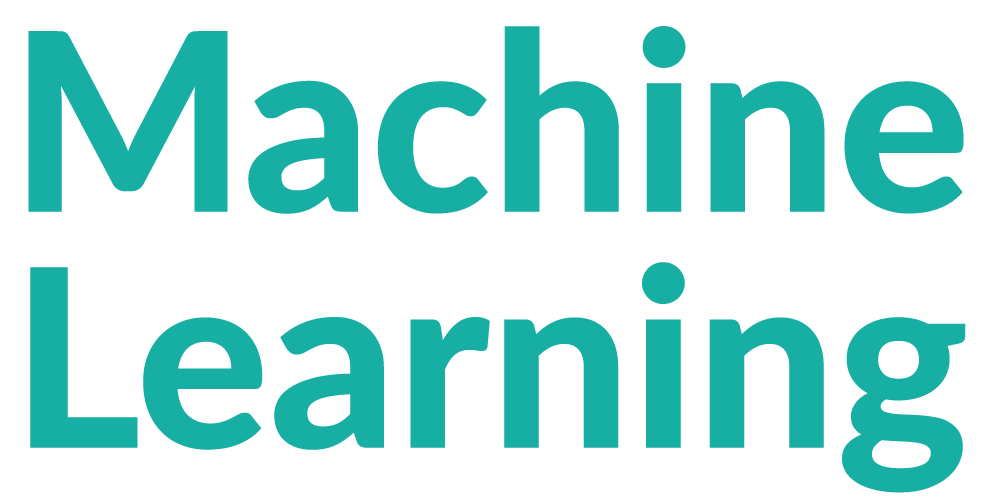
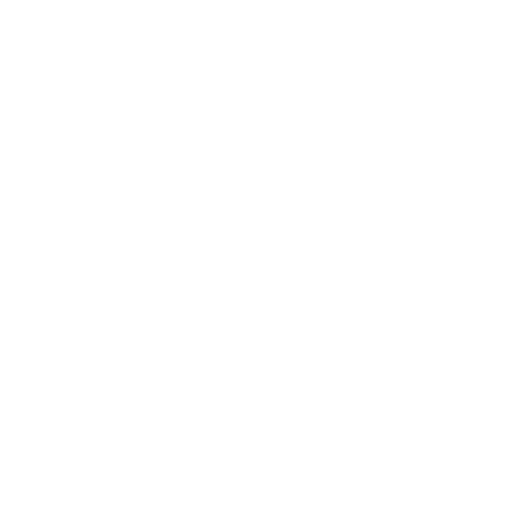
Machine learning is a branch of artificial intelligence (AI) and computer science which focuses on the use of data and algorithms to imitate the way that humans learn, gradually improving its accuracy.
Machine learning is important because it gives companies a view of trends in customer behavior and business operational patterns, as well as supports the development of new products. Many of today's leading companies, such as Facebook, Google, and Uber, make machine learning a central part of their operations. Machine learning has become a significant competitive differentiator for many companies.
Importance of Machine Learning in Business Intelligence
Business intelligence means the strategies and tech companies use to collect, interpret, and utilize data which plays an important role in briefing the strategies, functions, and efficiency of a company. However, as essential to a company's success as business intelligence is, many businesses don't take advantage of the tools that can improve their business intelligence efforts.
Machine learning is used to get insights from data and enables companies to understand the trends better and make weighted decisions. Business intelligence uses basic calculations to provide answers, while machine learning with predictive, prescriptive, and cognitive analytics uses mathematical models at this point of work, to determine attributes and offer prediction.
Business Intelligence has already moved away from long-outdated static reports to interactive dashboards and real-time analytics and allows businesses to have a descriptive vision based on the accumulated visual data. Machine learning algorithms, applied to datasets, can help to verify whether a hypothesis regarding the data is true or not. Basic machine learning algorithms can determine patterns in the data that would be beyond the bounds of identification using manual methods.
Combining machine learning with business intelligence can have a far-reaching impact on the insights a business gets from its available data, making business intelligence a true game-changer in helping companies improve productivity, quality, customer service and more.
Applications of Machine Learning in Business Intelligence
Following is some of the important applications of machine learning in business intelligence:
Improved Operational Processes
Machine learning can improve many operational processes, such as customer service, finance, marketing and much more. Machine learning can collect and use data from all these aspects of a business and help you automate processes to increase productivity. Machine learning can be integrated in a company’s ERP (Enterprise Resource Planning) system for a business to run more efficiently.
Personalized Customer Funnels
Business intelligence professionals don’t seem to realize that machine learning can seriously affect both the top and bottom of your customer funnel. As the importance of personalization on websites, email campaigns and even Facebook ads increases, it’s so important to be using machine learning to make your prospective customers feel important. Personalize your message for them at both the top and bottom of the funnel.
Better Customer Experiences
Machine learning gives business leaders the capability to analyze massive amounts of data and extract actionable insights in an instant. For customer service, this can be leveraged to change customer sentiment, detect dissatisfaction and fix any damaged relationships. For all businesses today that use machine learning, better customer experiences are just one algorithm away. Real-time chatbots are one of the foremost forms of a better customer experience.
Learn More About Each Prospect
Machine learning helps business intelligence professionals learn more about each prospect individually. Business intelligence professionals and their marketing counterparts can then activate that insight for each unique prospect to tailor their journey through the marketing channel and deliver more revenue. Machine learning can find patterns and help business intelligence and marketing leaders activate experiences at a level of granularity that was not possible before.
Analyze Large Sets of Data
Business Intelligence processes involve analyzing large sets of data and if done manually, this is time-consuming. Machine learning can automate the process so that business intelligence professionals can focus on higher-level trend analysis and behavior patterns that can bring greater value more quickly to the organization.
Improved Data Quality Checks
While it is common to use machine learning to predict and automate business decisions, machine learning can be used by business intelligence teams to improve the way they do data quality checks including both extraction and transformation. For example, anomaly detection in data, outlier identification and triaging, metadata checks and cataloging data better for use by business and analytics users. All these help business intelligence in improving data governance standards.
Provides Actual Forecasting Answers
Beyond demand models that only predict market trends, revenue levels and so on, machine learning can generate actual answers. As some businesses are now discovering, machine learning lets them produce highly accurate estimates of future behavior for instance; answers based on large volumes of historic data. Ongoing advances in neural networks also continue to aid the machine learning push, making business intelligence, and forecasting more intelligent and concrete.
Achieve Real-Time Data Analysis
With machine learning, anomalies can be spotted in real time and immediate action can be taken. For instance, fraud can be spotted right away and not a week later. Systems can be created immediately to avoid the anomalies in the future thus increasing the operational efficiency as well as making real-time decisions. Machine learning is assessing individual customer behavior as it happens. It is constantly analyzing ‘normal’ customer activity, so when it spots an anomaly, it can automatically block or flag a payment for analyst review.
Identify Patterns Among Employees
There is a lot of discussion in business intelligence regarding how product data can be used for growth, but not enough of it is related to the creation and development of the product. The same machine learning algorithms that detect patterns and anomalies within the market can be applied to your internal process for instance to analyze metrics like time spent on tasks, automate this analysis then use the data to become more efficient.
Build Optimum Data Pipelines
Machine learning can be used for business intelligence to analyze your source data and the underlying metadata in its native state, with the resulting data used to recommend and build the most optimum data pipelines and storage locations (based on data types, size, projected uses, etc.). It can suggest correlations between data elements for building recommendations on how to categorize and document the data.
Fight Cybercrime
In cybersecurity, automated protection to reduce risk windows or revenue loss is critical. This creates an enormous amount of data processing that must be analyzed quickly. Mature machine learning systems can autonomously collect, analyze, and classify threats. This ability to scale with machines is vital to fight cybercrime and helps reduce operating expenses and improve accuracy with continuous learning.
Create A Data Warehouse Cloud
Machine Learning can translate data concepts into business language and use that information to establish a data warehouse cloud. This can act as a semantic layer that maps business intelligence concepts within a data architecture, creating a credible and good-quality point of reference for the business. This will build a digital environment that understands your business and is better suited to answer specific questions.
Increase Productivity
Another great application of machine learning in business intelligence is analysis-ready data that allows for more proactivity while simultaneously setting achievable goals in future predictions, based on past data and not on traditional presumptions. So, the decision-making process is faster and the overall team function on outcomes is much more efficient.
Improved Financial Modeling
Applications of business intelligence in finance are pervasive and numerous. Financial modeling built on machine learning algorithms involves developing a mathematical model that translates the behavior of markets/agents into numerical predictions. For example, prescriptive analytics models aid with creating optimal portfolios or financial assets and putting together the optimal capital budgeting options.
Improved Risk Assessment
Risk assessment in business intelligence is important as it allows for a comprehensive analysis of possible issues associated with a business. Risk analysis using machine learning helps decision makers to estimate the impact of certain decisions and assess the value of a payoff that may occur. This is especially relevant when the problem being faced is an uncertain and risk-filled pattern of future events that often occurs in the financial realm. The aim of decision analysis is to determine the best decision alternative or the preferable decision strategy, utilizing data about uncertain events, possible consequences, and probable payoffs.
Customer Churn Modeling
Machine learning and artificial intelligence can also be used for business intelligence to identify when a customer’s loyalty is starting to diminish and to find strategies to resolve it. The organization can then use machine learning capabilities to evaluate patterns among existing customers to find out which customers are likely to abandon the business and go somewhere else, identify the reasons behind those customers’ decision to leave, and then determine the necessary steps the business should take to retain them.
Customer Recommendation Engines
Machine learning powers the customer recommendation engines built to deliver customized experiences and improve the overall customer experience. Here, algorithms analyze data points about each customer, including the customer’s previous purchases, and other data sets like demographic trends, an organization’s present inventory, and the purchase histories of other customers to know what services and products to offer as recommendations to each individual customer. A few examples of businesses whose enterprise models are based on recommendation engines: Amazon, Netflix, and YouTube.
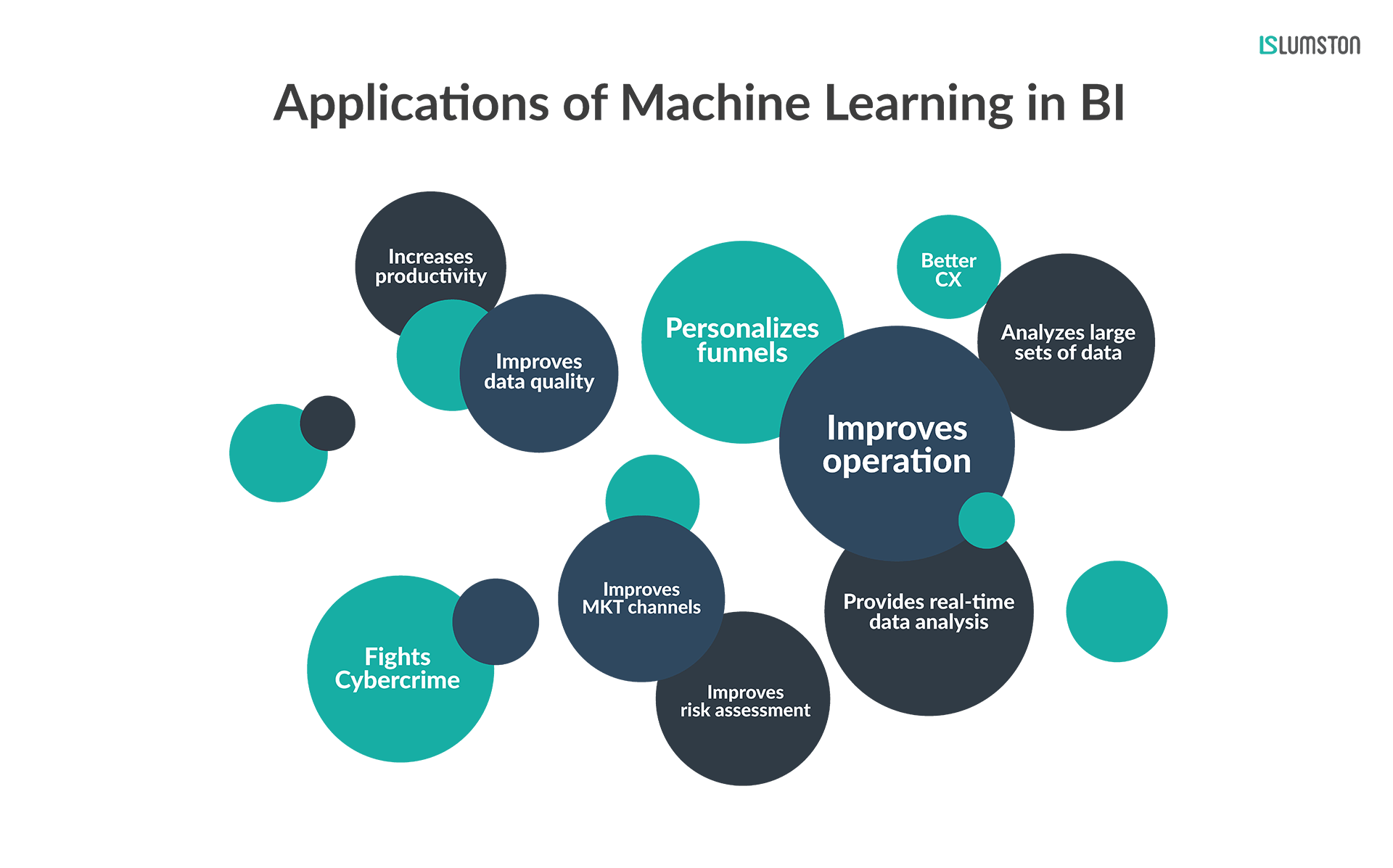
If you are planning to implement a Machine Learning in your company, feel free to contact us, just shoot an email to hello@lumston.com and we’ll get an expert into the conversation so we can help.