Data Lake Vs Data Warehouse - All You Need to Know!
Although both data warehouse and data lake are commonly applied for big data storage, the concepts are not identical. A vast collection of unstructured raw data is known as a data lake. A data warehouse, on the other hand, is a collection of processed data that has already been formatted, sorted, and structured for a particular purpose.
These two forms of data storage are frequently mixed together, but they differ greatly from one another. The only aspect they both have in common is the huge amount of data they possess.
That's why making the distinction between data warehouse and data lake is essential, as they have different uses and demand different methodologies for data optimization.
In this post, you'll get familiar with the concept of "data lake vs data warehouse" and their different roles.
So, without any delay, let's get straight into it!
Data Lake
Big data from multiple sources is found in a data lake in an unprocessed, natural manner, generally as files or objects. This central storage makes it possible for various datasets to store adaptable informational structures in huge volumes for later usage.
Simply keep your data in its current state, without any extra assembling, and do various analytics. These include real-time statistics, machine learning, big data dashboards, and visualizations, all of which are used to help people make wise choices.
Data Lake Pros
- Data lake emphasizes the rate of data entry, resulting in fast data loading.
- Data lake typically costs less than a data warehouse, making it easier to scale them as required.
- Data lake allows greater flexibility in how the data is eventually used because they keep the data in its raw state.
Data Lake Cons
- Real-time data lake querying is challenging and time-consuming.
- Due to data duplication and inconsistency, it may be vulnerable to reliability difficulties, making it more challenging to evaluate the data.
- A data lake can slow down assessment since it must first be cleaned and converted before it can be extracted and used for business purposes.
Data Warehouse
A data warehouse is a central storage for integrated data that can assist in making important choices when analyzed properly. Data is transferred from relational databases, transactional systems, and other systems until being cleaned up and checked before being put into a data warehouse.
Then, using SQL clients, business intelligence software, and other analytical programs, data analysts can retrieve this information. Statistics, dashboards, and advanced analytics are used often by many business divisions to make daily decisions within the entire firm. The two main methods for creating a data warehouse are (ELT (extract, load, transform) and ETL (extract, transform, load).
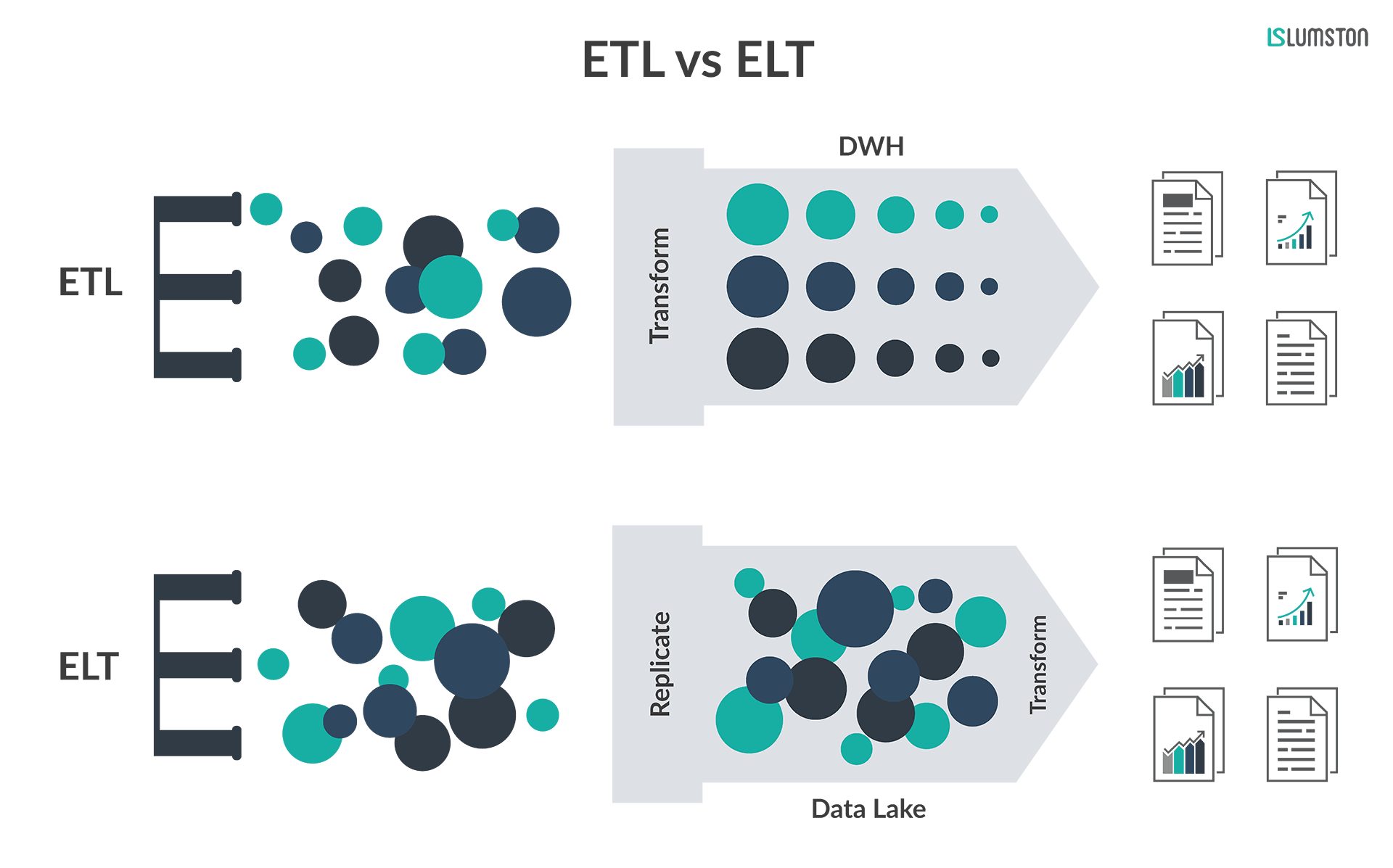
Data Warehouse Pros
- The speed of data extraction and evaluation is given priority in data warehouses as once the data is loaded, it can be queried and analyzed more quickly.
- You may be sure that analyses are built on consistent and reliable data since the information in your data warehouse has already been cleansed and organized.
- Analytics tools may easily link with structured data, making your data more comprehensible and accessible throughout the organization. Most of the time, SQL, which is quite popular, can be used to query the data.
Data Warehouse Cons
- Data warehouse is traditionally expensive, on-premises options. Although cloud data warehouse is altering that, costs may still rise as you scale.
- Front-loaded data cleaning and synthesizing require more work to enter and import data into data warehouses.
Data Lake Vs Data Warehouse - Top 6 Key Differences!
In the big data industry, there are many terminologies that every firm must be familiar with. A lot of these terms can be misunderstood quite easily just like data lake vs data warehouse.
What are some of their key distinctions, and how can your company use them to its benefit for data management and analytics?
Continue reading to find out how data lake and data warehouse differ from one another.
01 Support for Various Data Types
Web server logs, social network activity, sensor data, text, and multimedia files are a few great data lake examples. It implies that all of these can be supported by a data lake system. However, these non-traditional sources of data have also been ignored as accessing and storing them can be very costly and challenging.
On the other hand, a data warehouse typically contains the information that has been taken from transactional systems and is composed of quantitative measures and the attributes that characterize them.
02 Data Storage & Retention
Data analysts put in a lot of effort to study the data and determine how it can be used for business analysis before it can be deposited into a data warehouse. To facilitate the extraction of significant insights, they create transformations that summarize and modify the data. To save storage space and boost performance, a standard data warehouse is a costly and limited corporate resource. Information that doesn't directly address business issues must not be included in the data warehouse.
However, because all data, including raw, structured, or unstructured data can bevretained in a data lake architecture, data retention is less complicated. Since data can never bevlost, assessment of past, present, and future data facts is possible. The creation and scaling of data lakes to petabytes is quite simple as well. They don't have a storage cap because they operate on common systems and cheap storage resources.
03 Adaptability for Modifications
Due to the complexity of the data loading tasks and the effort put to make reporting and evaluation simple, a smart data warehouse architecture can adapt to change quite effectively. But developers will require a lot of time and money to make these modifications. Many businesses nowadays are concerned about how long it will take the data warehouse workforce to become familiar with their technology. Self-service business intelligence is a concept that has emerged because of time extension.
But with a data lake, all the data is kept in its original form and is constantly available to those who require it. Users are given the ability to study data in ways that go beyond what can be done in a data warehouse.
04 User Support
A data warehouse is a great solution for users that regularly review reports, assess key performance indicators, or organize datasets in spreadsheets. As a result, a data warehouse is suitable for "operational" workers because it is uncomplicated and was created with their needs in mind.
A data warehouse can assist users who perform more in-depth data analysis. For data integration, data analytics, and data preparation, they frequently turn to a data warehouse. The data warehouse can be used by people to perform detailed reviews, which may result in the creation of entirely new information sources based on the findings. These individuals are typically data analysts who employ great analytical techniques like statistical analysis and predictive modeling.
All these individuals are fully supported by the data lake architecture as well. Consider the scenario in which a data analyst can use their data lake system to deal with the very big and varied datasets they need, while their business customers can utilize a more insightful view of the data offered for their usage.
05 Utilization, Stability, & Security
Data warehouses are a trusted, enterprise technology that has been around for like twenty years. Although they are more recent and have a less extensive enterprise history, data lakes are still making progress. A large organization cannot simply purchase and build a data lake as it would a data warehouse. Rather, it must decide which technologies to utilize, commercial or open source, and how to combine them to satisfy client requirements.
Each technology has various end users. Business analysts utilize data warehouses to query the data using BI and pre-integrated reporting. Because data must be processed and analyzed to be usable, business users cannot employ a data lake as effectively. Big data in the data lake can be mined for insights by data scientists, data analysts, or experienced business users.
06 Quick Reviews & insights
Data lakes incorporate all data and data kinds, allowing users to access data prior to its transformation and structuring. As a result, users can access data more quickly than with a standardized data warehouse model.
This strategy might not be as practical as it seems, though. For some of the data sources used for analysis, the data warehouse team's regular tasks may differ from one another. However, a corporate user would not want to perform that duty, this will provide users the freedom to explore and employ the data as it is. The primary purpose of a business user's use case is to get reports and KPIs.
These operational reports will employ a more structured picture of the information with a data lake, which will support what they already had in the data warehouse. As opposed to physically inflexible tables that require a developer to alter, this method treats the data in the lake usually as metadata that lies above it.
Data Lake Vs Data Warehouse - Are Both Necessary?
You may be debating at this point whether your business needs both, a data warehouse,and a data lake.
The answer is: perhaps both!
While many businesses choose to combine data lakes and data warehouses as part of larger data architecture, the choice is usually influenced by the following factors:
- Analytics & Data Stack: You'll need a solution that effortlessly integrates with the other technologies you already regularly use.
- Data Access: A data lake can be appropriate if just members of the technical team require access. A data warehouse that integrates with tools made for corporate users is a better choice if users require to evaluate and query data.
- Data Utilization (Present & Future): Because the data stored there hasn't been converted into a certain format, data lakes seem more adaptable for a variety of use cases.
- Data Security: Cloud data warehouses guarantee that you completely control and own your data, and it's simpler to secure in accordance with regulatory standards.
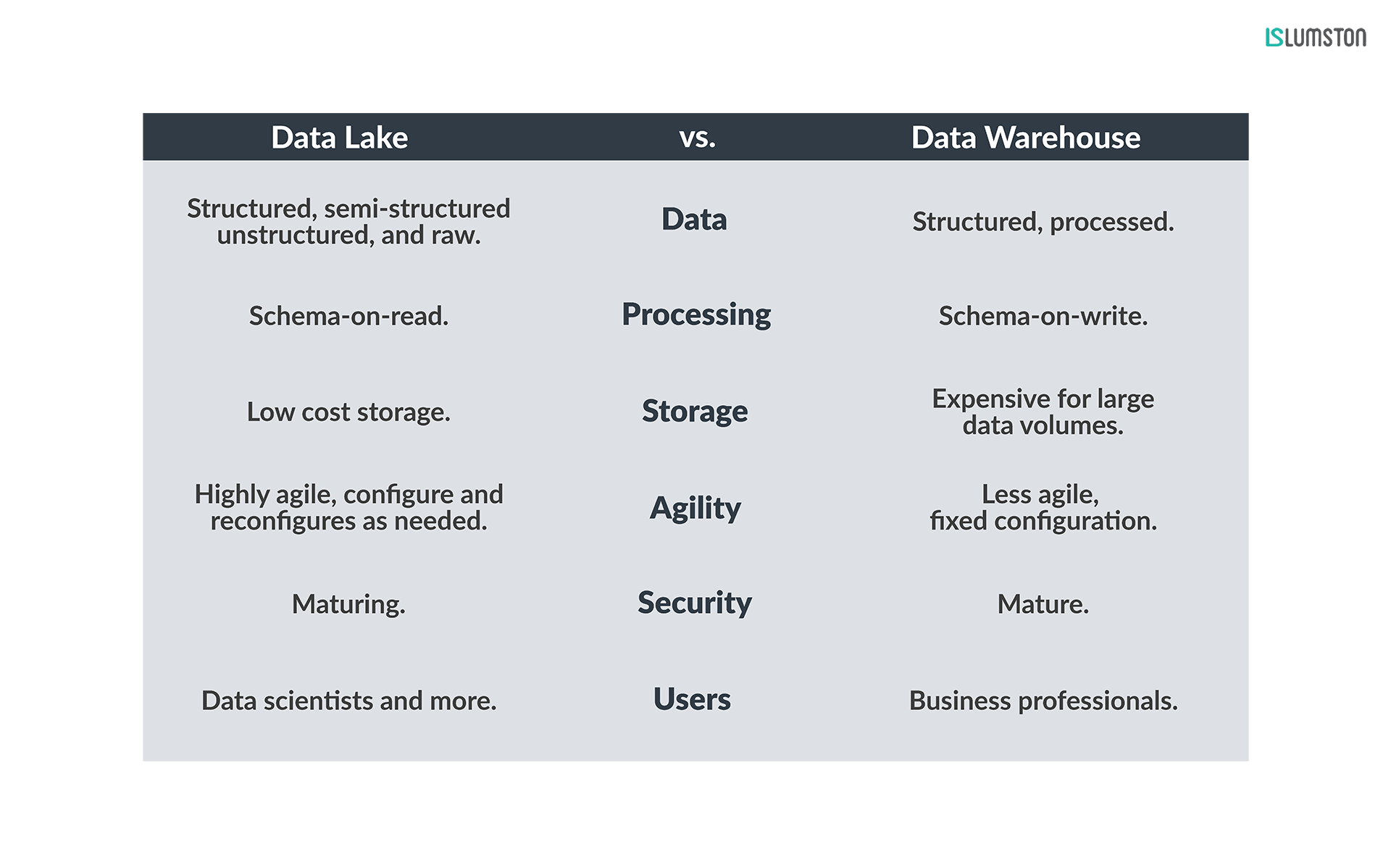
That’s all for Data Lake Vs Data Warehouse!
Now, that you’ve learned all the significant aspects of both data storage mechanisms, you’re all set to optimize your business cost!
If you are planning to implement a Data Lake or Data Warehouse in your company, feel free to contact us, just shoot an email to hello@lumston.com and we’ll get an expert into the conversation so we can help.
Lumston has been recently recognized by DesignRush as one of their recognized agencies on software development, learn more about Custom Software Development Cost.